bangladesh mask study: do not believe the hype
this is one of the worst studies i've ever seen in any field. it proves nothing apart from the credulity of many mask advocates.
people tend to call economics “the dismal science” because it’s so difficult to do controlled experiments. you cannot simply run the economy once with interest rates at 4% then again at 6% and compare. it’s inherently limiting to the discipline.
but this bangladesh study, originally published by “the national bureau of economic research” is so bad that i fear this is way past that. (newer version HERE but this the same study with new data cuts added.) even those legends of non-replication and bias injection in sociology and psychology would not accept a study like this. i have no idea how NBER fell for it.
(addendum: and they just published the new one)
this one would get you laughed out of a 7th grade science fair.
it violates pretty much every single tenet of setting up and running a randomized controlled experiment. its output is not even sound enough to be wrong. it’s complete gibberish.
truly, a dismal day for the dismal science and those pushing it into public health.
and yet the twitterati (and there are MANY) are treating this like some sort of gold standard study.
“This should basically end any scientific debate about whether masks can be effective in combating covid at the population level,” Jason Abaluck... who helped lead the study said... calling it “a nail in the coffin”
despite wild claims by the authors, it’s not.
this setup and execution is so unsound as to make it hard to even know where to start picking it apart, but it’s easy to throw shade and backing it up is the essence of debate and refutation, so let’s start with the basics on how to do a medical RCT.
establish the starting condition. if you do not know this, you have no idea what any later numbers or changes mean. it also makes step 2 impossible.
randomize cohorts into even groups in terms of start state and risk. this way, they are truly comparable. randomizing by picking names out of a hat is not good enough. you can wind up doing a trial on a heart med where 20% of active arm and 40% of control have hypertension. you cannot retrospectively risk adjust in a prospective trial. this needs to be done with enrollment and study arm balancing. fail this, and you can never recover.
isolate the variable you seek to measure. to know if X affects Y, you need to hold all other variables constant. only univariate analysis is relevant to a unitary intervention. if you give each patient 4 drugs, you cannot tell how much effect only drug 1 had. you’ve created a multivariate system. get this wrong, and you have no idea what you measured.
collect outcomes data in a defined, measurable, and equal fashion. uniformity is paramount. if you measure unevenly and haphazardly, you have no idea what manner of cross confounds and bias you have added. you will never be able to separate signal from measurement artifacts. this means your data is junk and cannot support conclusions.
set clear outcomes and measures of success. you need to decide these ahead of time and lay them out. data mining for them afterwards is called “p-hacking” and it’s literal cheating. patterns emerge in any random data set. finding them proves nothing. this is how you perform a study that will not replicate. it’s a junk analysis.
OK. there’s our start point for an RCT.
get any one of these steps wrong, and your study is junk. get several wrong and your study is gibberish. get them ALL wrong, and you’re either using data to mislead or you really have no business running such studies.
now let’s look at what was done in bangladesh:
establish starting condition and balance cohorts: this was a total fail and doomed them before they even began.
this is their key claim in the finding. but notice what is jarringly absent: any clear idea what prior disease exposure was in each of these villages and village cohorts at study commencement. small current seroprevalence probes cannot tell us this. there is no way to know if one had had a big wave and another had not. so, we literally have no idea what had happened here and on what sort of population. we have no idea if we are studying a naïve population or not.
performing this study without a clear and broad based baseline and sense of past exposure is absurd. this is a tiny signal (7 in 10,000) we need a very high precision in start state. it’s absent. that’s the ballgame right there. we do not know and can never know what happened. even miniscule variance in prior exposure would swamp this.
for cohorts, they paired by past covid cases (so thinly reported as to be near meaningless and a possible injection of bias as testing rates may vary by village) and tried to establish a “cases per person” pair metric to sort cohorts. this modality is invalid on its face without reference to testing levels. you have no idea if high cases are high testing or high disease prevalence. you have no idea how much testing varied.
and testing levels are so low, the authors estimate a 0.55% case detection rate. so a modality invalid even if data were good is multiplied in terms of error because the data is terrible (and likely wildly variable by geography)
nowhere here does the word “testing” or “sample rate” appear.
so this study ended before it even began.
this was not useful randomization. this was garbage in garbage out especially when you later seek to use “symptomatic seroprevalence” as primary outcome. that’s false equivalence. if you’re going to use seroprevalence as an outcome, you need to measure it as a start state and balance the cohorts using it. period. failure to do so invalidates everything. you cannot run a “balance test” on current IgG and presume you know what happened last year, certainly not to the kind of precision needed to find a 0.0007 signal meaningful.
this was an unknown start state in terms of highly relevant variables and the cohorts were not normalized (or even measured) for it.
they also failed to measure masked vs unmasked seroprev in any given village. that would have been useful control data. it seems like they really just missed all the relevant info here.
it’s pure statistical legerdemain.
strike 1 and 2.
but it gets worse. much worse.
isolate the variable you seek to measure.
to claim that masks caused any given variance in outcome, you need to isolate masks as a variable. they didn’t. this was a whole panoply of interventions, signage, hectoring, nudges, payments, and psychological games. it had hundreds of known effects and who knows how many unknown ones.
we have zero idea what’s being measured and even some of those variables that were measured showed high correlation and thus pose confounds. when you’re upending village life, claiming one aspect made the difference becomes statistically impossible. the system becomes hopelessly multivariate and cross-confounded.
the authors admit it themselves (and oddly do not seem to grasp that this invalidates their own mask claims)
who knows what the effects of the “intervention package” were outside of masking? maybe it also leads to more hand washing or taking of vitamins. it seemed to effect distancing (though i doubt that mattered).
but the biggest hole here is that that which affects one attitude can affect another.
if you’ve been co-opted to “lead by example” and put up signage etc or are being paid to mask you may change your attitude about reporting symptoms.
people want to please researchers and paymasters and this is a classic violation of a double blind system. the subjects should no know if they are control or active arm, but in the presence of widespread positive mask messaging, they do.
so maybe they think “i don’t not want to tell them i’m sick.” especially if “being sick” has been vilified.
or maybe they fail to focus on minor symptoms because they are masked and feel safe or were having more trouble breathing anyhow.
this makes a complete mess and injects all manner of unpredictable bias into the “results” because the results are based on “self reporting” a notoriously inaccurate modality.
look at the wide variance in self reporting on masks and reality cited in this very study.
yet we’re to accept self reporting of symptoms in the face of widespread and persistent moral suasion in one arm and not the other and assume that the same interventions that had a large effect on mask wear affected no other attitudes?
no way. this non-blinded issue combining with self reporting adds one tailed error bars so large to this system that they swamp any signal.
as is so often the case, gatopal @Emily_Burns-V has a great take here:

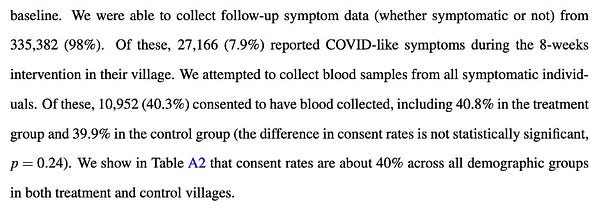

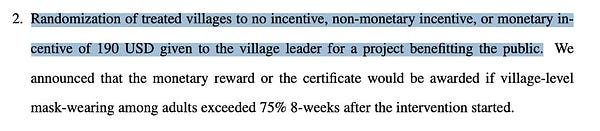
this is exactly why good studies are blinded. if they are not, the subjects seek to please the researchers and it wrecks the data. adding self reporting is a multiplier on this problem. this whole methodology is junk and so is the data it produced.
for proof of this, one need only look at the age stratification:
first off, this proves conclusively that “your mask does not protect me.” (though we already knew that) if it did, it would protect everyone, not just old people. but it didn’t. and the idea that it stopped old people from getting sick but not young people is similarly implausible.
the odds on bet here is that old people were more inclined to please the researchers than young people and that they failed to report symptoms as a result.
i establish this as the null hypothesis.
can anyone demonstrate that this data makes a more compelling case for “masks worked on old people but not young people and thus decreased overall disease”?
because i very much doubt it.
and unless you can, you must abandon this study as a possibly interesting piece of sociology, but as having zero validated epidemiological relevance.
so, that’s strikes 3 and 4.
i’m honestly a bit unsure about whether we can go on to call it a perfect 5. they did pre-register the study and describe end states, but they never established start states so we have no idea what actual change was.
so, they called their end results shot beforehand (as they should) but then left us with no way to measure change even if the measurement was good, and as we have seen, the measurement was terrible.
so i’m going to sort of punt on scoring this one and assign it an N/A. establishing an outcome and then providing no meaningful way to measure it is not p-hacking per se, but it is also not in any way useful.
so, all in all, it’s just impossible to take this study seriously, especially as it flies in the face of about 100 other studies that WERE well designed.
read many HERE including RCT’s showing not only a failure as source control, but in higher rates of post op infections from surgeons wearing masks in operating theaters vs those that did not.
the WHO said so in 2019.
and the DANMASK study in denmark was a gold standard study for variable isolation and showed no efficacy.
perhaps most hilariously, the very kansas counties data the CDC tried to cherry pick to claim masks worked went on to utterly refute them when the covid surge came.
the evidence that masks fail to stop covid spread is strong, deep, wide, and has a lot of high quality studies. (many more here from the swiss)
to refute them would take very high quality data from well performed studies and counter to the current breathless histrionics of masqueraders desperate for a study to wave around to confirm their priors, this is not that.
to claim “masks worked” with ~40% compliance in the light of total fails with 80-95% is so implausible as to require profound and solid evidence that is nowhere provided here.
this is a junk output from a junk methodology imposed upon an invalid randomization without reference to a meaningful start state for data.
this is closer to apples to orangutans than even apples and oranges.
thus, this and many more like is an absurd and impossible take from this study.
it does not show efficacy. it does not show ANYTHING outside of how an object lesson in poor study design and data collection can be weaponized into a political talking point.
this study is an outright embarrassment and a huge black eye for the NBER et al.
this is not even wrong.
it’s just an epic concatenation of bad techniques and worse data handling used to provide pretext for an idea the researchers clearly favored. there is no way to separate bias from fact or data from artifact.
calling this proof of anything is simply proof of either incompetence or malfeasance.
which one makes you want to listen to the folks pushing it?
Gato 🐱 I know you are not a doctor, but somehow you know your stuff well about what is important in a RTC. Everything you say is true; it is a crap study. But I will add one more strike. Even IF they had done a decent study, the differences they found are so minuscule and unimportant... It is a classic example of findings that reach statistical significance (p<0.05) due to a very large sample size. But if the numbers were true, they effect would be so small that it would certainly not justify requiring everyone in the population to wear a frigging mask. (How many strikes did they get? I thought three strikes and you are out).
Well … another data point in the soon to be written barn burner titled, “The End of Enlightenment: Our New Dark Age.”
1. Pick a side on a contested issue.
2. Fund a crap study that will hopefully provide numbers that support your side on the policy issue.
3. Publish the crap study in a journal of a field that gave us the last global financial crisis (aka a hack field).
4. Authors of crap study go on twitter and declare that the science is settled.
5. Journos, who now can only stay focused long enough to read a tweet, write a story that the “science is settled.” and link to said author tweet as proof.
6. The “wires” spread journos' “report” hither and yon.
7. TV “experts” read the report from the wires and then go on TeeVee to tell viewers that “the science is settled.”
8. Policy makers and politicians watch the TV experts pronouncement, and proceed to enact said policy because … “the science is settled.”
9. The lights start going out in cities across the land.